The high mortality rate of COVID-19 is largely due to acute respiratory distress syndrome (ARDS), which is characterized by diffuse alveolar damage (DAD). Additionally, severe cases of COVID-19 often result in a cytokine storm and a disrupted adaptive immune response. Most previous studies on this issue have focused on the peripheral cell count and the functionality of immune cells.
The team at UK Augsburg in Germany used multiplexed immunofluorescence to study the impact of SARS-CoV-2 on antigen-presenting cells. Like MERS-CoV and SARS-CoV, SARS-CoV-2 appears to impair the maturation of dendritic cells (DCs), which is characterized by a switch in surface antigen expression. This switch enables the cells to travel to lymph nodes and activate T-cells.
To shed light on the local inflammatory infiltrate, the team compared the cell populations of professional antigen-presenting cells (APCs) in the lungs of COVID-19 autopsy cases in various stages of DAD. They found an increased number of myeloid dendritic cells (mDCs) in later stages, but with no significant upregulation of maturation markers in DAD specimens with high viral load. This accumulation of immature mDCs, which are unable to reach lymph nodes, results in an inadequate T-cell response.
Overall, this study highlights the need for further research to understand the impact of SARS-CoV-2 on the immune system and to develop more effective treatments for COVID-19Discover how the team employed digital analysis of multiplex IF images to gain insights into the unique immune profile in fatal COVID-19 cases. Investigate the potential impacts of SARS-CoV-2 and related coronaviridae on antigen-presenting cells. Learn about the role played by the UKA in COVID-19 autopsies.
-
- Experience how the team used digital analysis of multiplex IF images to learn more about the specific immune landscape in fatal COVID cases
-
- Explore the possible effects of SARS-CoV-2 and related coronaviridae on antigen presenting cells
-
- Understand the role of the UKA in COVID-19 autopsies
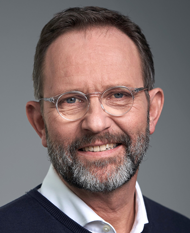
Prof Ralf Huss, University Hospital Augsburg
Ralf Huss, MD, PhD, is a Professor of Pathology and the Managing Deputy Director of Pathology and Molecular Diagnostics at the University Hospital in Augsburg, Germany. He is also the head of the Center for Digital Medicine.
Huss holds board certifications in anatomical, experimental, and molecular pathology and boasts over 30 years of expertise in histopathology, immunology, cancer research, and oncology.
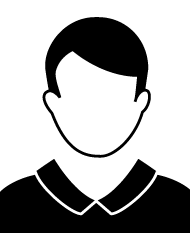
Lukas Borcherding, Medical Student, Technical University of Munich
Lukas Borcherding is a trained nurse who has been pursuing a degree in medicine at the Technical University of Munich, Germany since 2015. In 2020-2021, he took a hiatus from his studies to concentrate on the COVID-19 pandemic and examine the unique immune profile in fatal cases. Following the completion of his project, Lukas has resumed his studies and is presently finishing the practical component of his education. Upon receiving his license, he intends to work in internal medicine.
-
- Discover how the Visiopharm platform can be used for multiplex analysis
-
- Review an example analysis of an mIF 8plex image
-
- Learn how the new Visiopharm Data Insights tool can be used for exploring image objects
-
- See an example analysis of a Fluidigm Hyperion CyTOF image covering tissue and cell segmentation using AI deep learning, phenotyping and an example tumor microenvironment region analysis
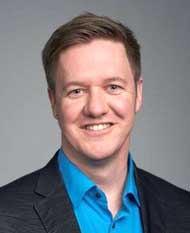
Dr Fabian Schneider, PhD, Service Project Lead, Visiopharm
Dr Fabian Schneider is part of Visiopharm’s R&D and Product Management team, responsible for phenotyping products as well as service projects for custom APP development. Fabian has over 10 years of international experience in cancer biology and immuno-oncology, working in academic research labs, clinical research teams and computational pathology groups in both academia and biopharma. Fabian received his Dr phil. nat. in Cell Biology in 2011 from the Johan Wolfgang Goethe University Frankfurt, Germany.


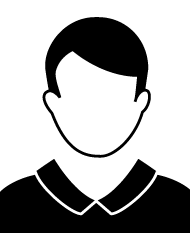
Joseph Allison, MSci, Research Technician | UK Dementia Research Institute, King’s College London
Joseph Allison MSci, is a Research Technician at the UK Dementia Research Institute, King’s College London. He studied and received his MSci degree from King’s College London with two extended research projects involving behavioural assays in Drosophila to elucidate the development of ‘negative’ associative learning as well as AAV stereotaxic injections in mice to determine the neural connectivity underpinning sunlight-mediated mood and cognition. In 2019, he joined the Christopher Shaw Lab with the team’s research focus centred around gene therapy and pathomechanisms in amyotrophic lateral sclerosis and frontotemporal dementia.
Over the last two years he has worked in the histology team processing animal tissue for immunohistochemical and fluorescent staining with subsequent imaging on a slide scanner or confocal, multiphoton, and calcium imaging microscopes. He is now in charge of quantifying the images obtained using software such as Visiopharm to develop apps informing business decisions through analysis of efficacy and toxicity.
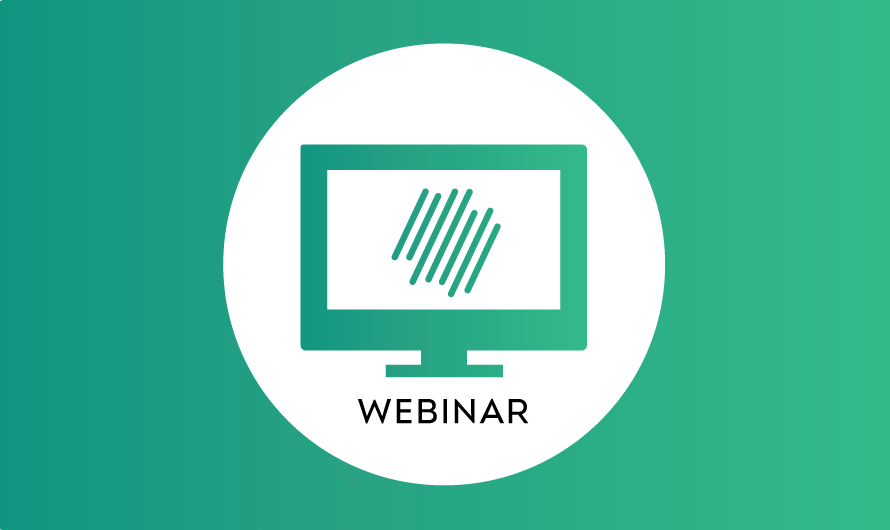
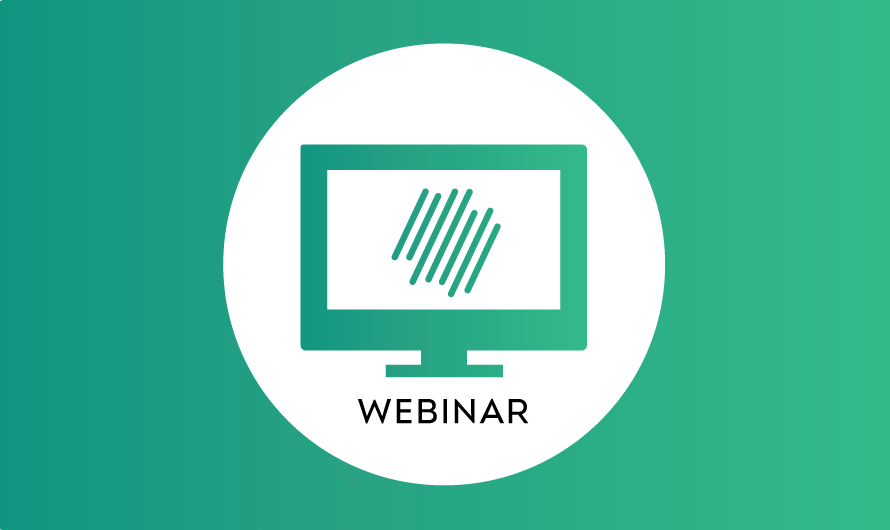
Quantitative histological analyses are used to infer pathological diagnoses and identify biological drug targets in human and laboratory animal samples. Each histological sample often include millions of cells imposing a need for automated unbiased quantitative analysis to replace manual assessment.
We have trained a convolutional neural network that can identify individual nuclei inferred from DAPI fluorescence, allowing automated quantitative analysis of various fluorescent marker levels in nuclei. We used this convolutional network to assess proliferation from nuclear Ki67 fluorescence and apoptotic DNA fragmentation from terminal deoxynucleotidyl transferase dUTP nick end labelling (TUNEL) at a single cell level in human kidney biopsies. Upon retraining, the convolutional neural network was adaptable to identify nuclei from pancreas emphasizing the versatility of the network. Taken together, these results demonstrate an AI-based automated method for quantifying fluorescence levels of various markers in diverse tissues such as kidney and pancreas.
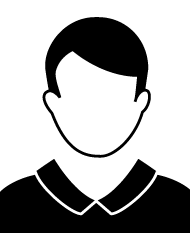
Tobias Højgaard Dovmark, Research Scientist, Novo Nordisk
As a research scientist at Novo Nordisk, Tobias Højgaard work with image analysis of histological samples. He has previously worked for Visiopharm as an image analysis specialist. He has a PhD in Physiology, Anatomy and Genetics from University of Oxford and an M.Sc. in Molecular Biomedicine from the University of Copenhagen.
Introduction: Liver biopsy is the gold standard to diagnose non-alcoholic Fatty Liver Disease (NAFLD) and the more progressive form steatohepatitis (NASH), and is the primary outcome in clinical trials for NAFLD treatment. The diagnosis is confirmed using the histopathological NAFLD-activity score (NAS), which grades the severity of steatosis, hepatocellular ballooning degeneration, and inflammation. Histopathological disease scoring systems are, however, subjective and prone to inter- and intra-observer variation. We therefore apply a deep learning image analysis strategy to obtain a more accurate and objective method for staging NAS in mouse models of NAFLD.
Materials and Methods: Using the Artificial Intelligence (AI) Deep Learning software from Visiopharm, the strategy was to perform a segmentation of liver biopsy sections stained for H&E from diet-induced obese (DIO) NASH mouse models and chow-fed controls. Inflammatory cells, lipid droplets, ballooned hepatocytes and hepatocytes with and without steatosis were annotated in H&E sections. Moreover, central veins and portal tracts were annotated. Thereafter, segmentations were postprocessed into the corresponding histopathological scores, and in addition quantitative measures of inflammation and steatosis were obtained.
Results: AI Deep Learning applications successfully recognized inflammatory cells, hepatocytes with and without steatosis, and ballooned hepatocytes in DIO-NASH mice. The app performance was comparable to manual evaluation of NAS, and confirmed significant improvement of NAS after 12w treatment with semaglutide in a DIO-NASH mouse model.
Discussion and Conclusion: We here demonstrate a deep-learning based approach to obtain the NAS scores in translational obese mouse models of DIO-NASH. A deep-learning approach for pattern recognition allows rapid and reproducible quantification of histological NASH parameters.
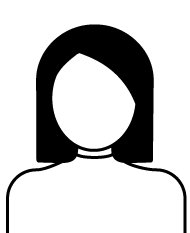
Agnete Overgaard, Research Scientist, Gubra
Agnete Overgaard has worked with Gubra for the past three years as a research scientist in the Tissue Research department. In this role she is responsible for processes ranging from optimizing protein and mRNA visualisation in tissue preparations, all the way to image analysis and histopathological evaluation of biopsy preparations from Gubra’s diverse animal models. Gubra is a biotech company that offers contract research services within the metabolic space, including extensive histology data packages. Over the years she has been involved in digitalization of workflows from biopsy to graph, including the development of several new image analyses. Recently, she was involved in the development of deep learning-based histopathological scoring of non-alcoholic fatty liver disease, that enables objective evaluation along with quantitative outputs.
She was previously a postdoc within the neurobiology field at University of British Columbia and Copenhagen University Hospital Rigshospitalet, and she holds a PhD in Neuroscience from the University of Copenhagen.
The immune response is spatially and temporally regulated. The density and location of immune cells in the tumor microenvironment (TME) have important diagnostic and prognostic values. Single cell-based multiomic technologies have exponentially increased our understanding of the numerous cellular and molecular networks regulating tumor initiation and progression. However, these techniques do not provide information about the spatial organization of cells or cell-cell interactions. Affordable, accessible, and easy to execute multiplexing techniques that allow spatial resolution of immune cells in tissue sections are needed to complement single cell-based high-throughput technologies.
We have developed a strategy that integrates serial imaging, sequential labeling, and image alignment to generate virtual multiparameter slides of whole tissue sections. Virtual slides are subsequently analyzed in an automated fashion using the VIS software allowing us to identify, quantify, and map cell populations of interest. Specifically, the image analysis is performed using the analysis modules Tissuealign, Author, and HISTOmap. Here, we propose a strategy for the rational design of tissue multiplex assays using commercially available reagents, affordable microscopy equipment, and user-friendly software. Using this strategy, we created one virtual slide comprising 11 biomarkers plus two frequently used histological stains: hematoxylin and eosin (H&E) and picrosirius red (PSR). Multiple immune cell populations were identified, located, and quantified in different tissue compartments and their spatial distribution resolved using tissue heatmaps. This strategy maximizes the information that can be gained from limited clinical specimens and is applicable to formalin-fixed paraffin-embedded (FFPE) archived tissue samples, including whole tissue, core needle biopsies, and tissue microarrays. We propose this methodology as a useful guide for designing custom assays for identification, quantification, and mapping of immune cell populations in the TME.
Presented on March 22, 2021 at an XTalks webinar.
-
- Integration of serial imaging, sequential labeling, and image alignment in the experimental design of imaging assays can greatly increase the number of markers that can be visualized simultaneously, expand the possibilities of the analysis, and extract more information from precious clinical specimens.
-
- Virtual multiplexing allows to determine how markers visualized in one section spatially relate to markers visualized in another contiguous section.
-
- The use of whole tissue sections instead of selected fields of view for the analysis, results in an unbiased representation of the TME.
-
- The use of tissue heatmaps greatly simplify the visual representation of the spatial organization of cells in the tissue.
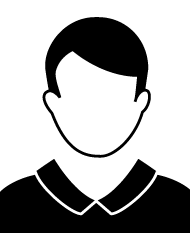
Manuel Flores, Ph.D. Candidate
Manuel Flores obtained his Biochemistry degree from Havana University, Cuba. In 2015 Manuel enrolled in the Immunology and Virology Master Program at Université de Montréal (Canada) and fast tracked to the Immunology and Virology PhD Program in 2016.
His doctoral research project focuses on characterizing the liver resident and infiltrating immune cell populations and their role in the pathogenesis of chronic liver diseases due to persistent viral and toxic injuries, including fibrosis and hepatocellular carcinoma. His research interests center around the spatial organization of immune cells in the hepatic tissue microenvironment, and the delineation of the multiple cell-cell interactions and their respective biological significance in health and disease. Manuel Flores is the recipient of doctoral scholarships from University of Montreal and from Fonds de recherche du Québec – Santé (FRQS).