This blog post is inspired by the scientific poster presented at the Pathology Visions conference in 2021 by Daniel E. Winkowski, Ph.D., Jenifer Caldara, Brit Boehmer, Ph.D., and T. Regan Baird, Ph.D.
Today, let’s explore three different approaches to segmenting cells in samples stained with various multiplexed fluorescent assays.
Starting with the challenge: Precision in Complexity
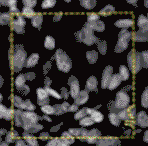
In tissue pathology, multiplex tissue images have become crucial, allowing us to observe each cell’s location and understand its complex features. Yet accurately and automatically defining each cell’s outer boundary for analysis proves challenging. The reason for that is that tissues contain a heterogeneous mix of cells, each of their own shape and size. Commonly used methods for cell segmentation only focus on nuclei, but those often fall short in precisely defining cell edges.
Let’s compare the methods: Traditional vs. A.I.-Powered
In this study, Daniel Winkowski and team used Visiopharm’s image analysis software to explore three different approaches to segment cells in samples stained with different multiplexed fluorescent assays:
1. Traditional Image Analysis (Trad): This method uses built-in filtering to enhance objects of interest, relying more on morphometry than intensity. While effective in identifying nuclei based on shape, it’s time-consuming and can struggle with diverse nuclear morphologies.
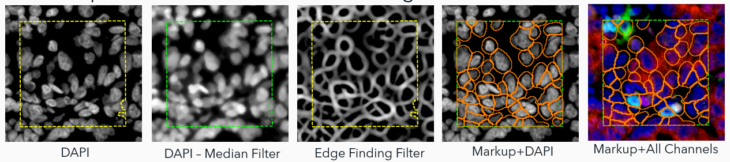
2. Ready-To-Use Deep Learning (AI): This approach uses a deep learning algorithm for nuclear signals identification and segmentation. It estimates cell boundaries based on dilation – a no-hassle method but still an estimation. This approach can underperform in identifying cells with clear biomarker staining but lacking a clear nucleus in the plane of section (i.e., CD68 macrophages).
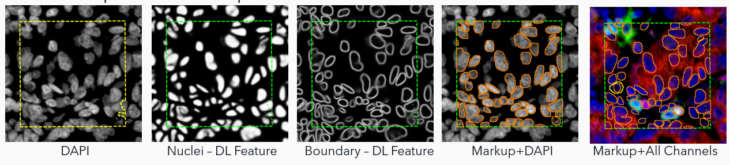
3. Adapted Deep Learning (AI+): The real star of the show! This method augments the deep learning algorithm to include signals from biomarker channels, assisting in accurately delineating cell boundaries. This blend of nuclei and biomarkers, coupled with an edge-finding filter, offers a more precise approximation of the cell border and assists in finding complex cells (i.e,. macrophages, etc.) where nuclear identification methods fall short.
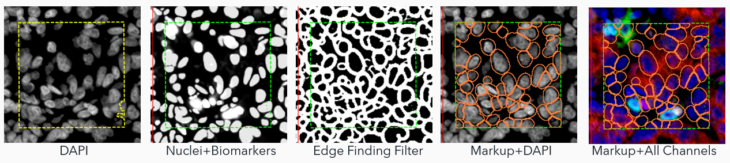
Visiopharm offers a complete toolbox for design and customizing your own algorithm but also offers a general nuclear detection algorithm which uses Deep Learning AI to segment nuclei of most morphologies (Ready-to-use AI approach).
To create the AI+ approach, the team adapted the ready-to-use algorithm to combine its nuclear AI classifier with signals from biomarkers in the multiplex panels to expand the segmentation beyond the nuclei, to the outer cell periphery.
The performance of these approaches was quantified by comparing them with manual annotations as a ground truth.
Ground truth annotations were prepared by three human observers on images from two different instrument manufacturers: Vectra Polaris 8-plex lung cancer (Akoya Biosciences) and Hyperion 13-plex spleen (Standard BioTools).
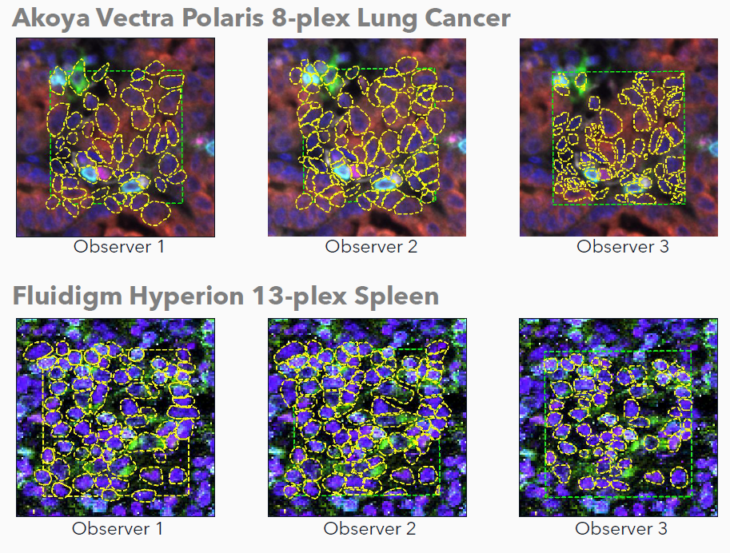
Manual annotations were prepared by three unique human observers on images from two different instrument manufacturers. Human observers utilized both nuclear and biomarker signals to aid during annotation placement. Note the variability across observers.
The AI’s performance was assessed against the ground truth annotations using Precision (a ratio of true and false positives), Sensitivity (a ratio of true positives and false negatives), and DICE Score (a ratio of all true/false, positive/negatives).
Results: AI+ Takes the Lead
After rigorous testing against manual annotations (the ground truth), the results were clear. While all algorithms performed admirably, the AI+ method showed the highest concordance with ground truth annotations. This means a more complete and accurate segmentation of cells across varying multiplex images.
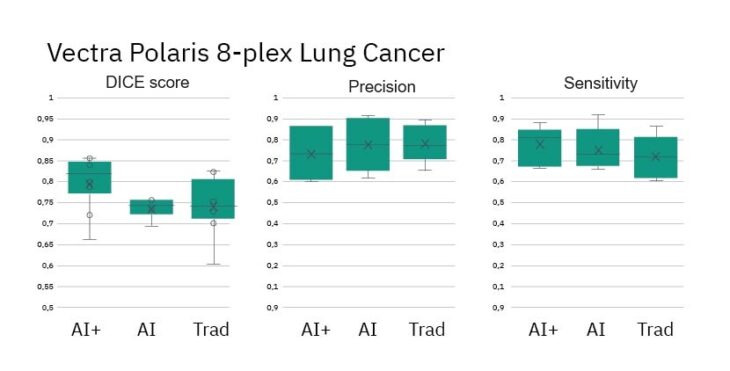
- DICE Scores for AI+ method show improved performance vs Ground Truth
- Precision and Sensitivity (measures of object detection) are similar across methods
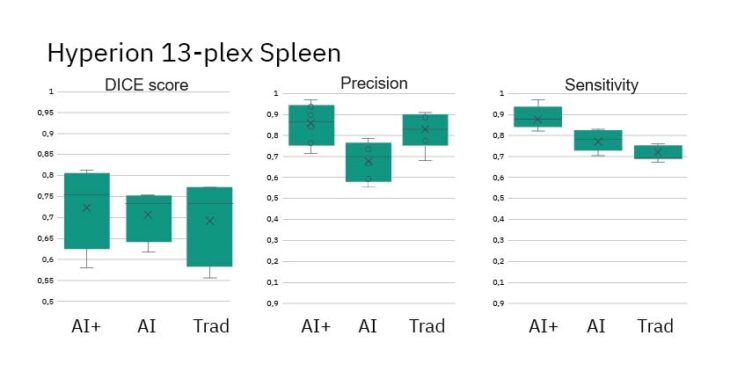
- DICE Scores for all methods show similar performance vs Ground Truth
- Precision and Sensitivity (measures of object detection) are roughly equal across methods
The Visiopharm has developed a flexible, AI-based strategy enabling comprehensive cell segmentation across various multiplex images.
- All algorithms performed at a high-level using industry standards including DICE Scores, Precision and Sensitivity.
- The Adapted Deep Learning algorithm showed highest concordance with Ground Truth annotations.
- Performance differences across instruments could be attributed to resolution differences of the instruments, the number of biomarkers in the panel, and differences in images tissue and disease state.
- Despite annotation differences among human observers, the algorithms perform consistently across the image set.
Would you like to see how Visiopharm’s software could support your cell segmentation challenges in multiplexed images? Learn more about Phenoplex, a complete workflow for all your multiplexed image analysis needs.
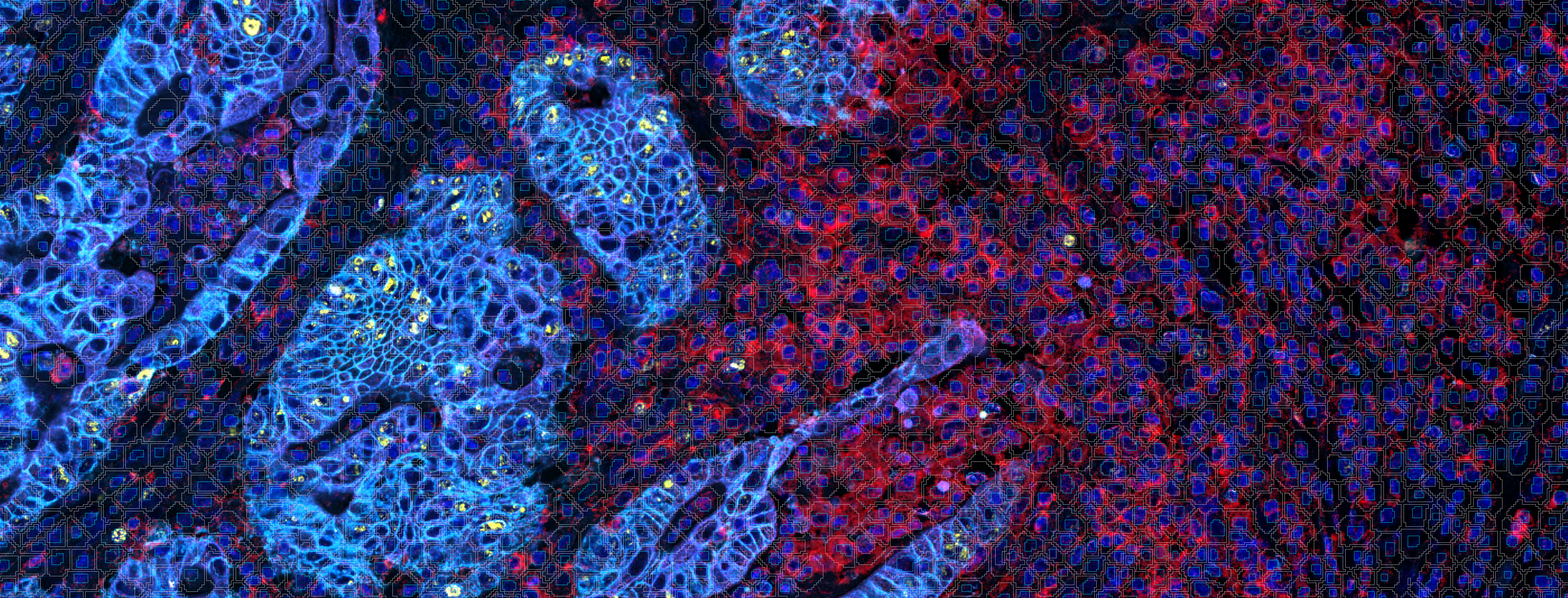