Introduction: Liver biopsy is the gold standard to diagnose non-alcoholic Fatty Liver Disease (NAFLD) and the more progressive form steatohepatitis (NASH), and is the primary outcome in clinical trials for NAFLD treatment. The diagnosis is confirmed using the histopathological NAFLD-activity score (NAS), which grades the severity of steatosis, hepatocellular ballooning degeneration, and inflammation. Histopathological disease scoring systems are, however, subjective and prone to inter- and intra-observer variation. We therefore apply a deep learning image analysis strategy to obtain a more accurate and objective method for staging NAS in mouse models of NAFLD.
Materials and Methods: Using the Artificial Intelligence (AI) Deep Learning software from Visiopharm, the strategy was to perform a segmentation of liver biopsy sections stained for H&E from diet-induced obese (DIO) NASH mouse models and chow-fed controls. Inflammatory cells, lipid droplets, ballooned hepatocytes and hepatocytes with and without steatosis were annotated in H&E sections. Moreover, central veins and portal tracts were annotated. Thereafter, segmentations were postprocessed into the corresponding histopathological scores, and in addition quantitative measures of inflammation and steatosis were obtained.
Results: AI Deep Learning applications successfully recognized inflammatory cells, hepatocytes with and without steatosis, and ballooned hepatocytes in DIO-NASH mice. The app performance was comparable to manual evaluation of NAS, and confirmed significant improvement of NAS after 12w treatment with semaglutide in a DIO-NASH mouse model.
Discussion and Conclusion: We here demonstrate a deep-learning based approach to obtain the NAS scores in translational obese mouse models of DIO-NASH. A deep-learning approach for pattern recognition allows rapid and reproducible quantification of histological NASH parameters.
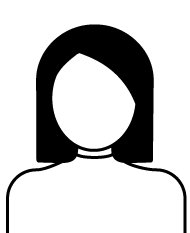
Agnete Overgaard, Research Scientist, Gubra
Agnete Overgaard has worked with Gubra for the past three years as a research scientist in the Tissue Research department. In this role she is responsible for processes ranging from optimizing protein and mRNA visualisation in tissue preparations, all the way to image analysis and histopathological evaluation of biopsy preparations from Gubra’s diverse animal models. Gubra is a biotech company that offers contract research services within the metabolic space, including extensive histology data packages. Over the years she has been involved in digitalization of workflows from biopsy to graph, including the development of several new image analyses. Recently, she was involved in the development of deep learning-based histopathological scoring of non-alcoholic fatty liver disease, that enables objective evaluation along with quantitative outputs.
She was previously a postdoc within the neurobiology field at University of British Columbia and Copenhagen University Hospital Rigshospitalet, and she holds a PhD in Neuroscience from the University of Copenhagen.