Resources / Improving Bladder Cancer Grading with AI-Enabled Computer Vision Externally Validating Grading Models and Incorporating Highly Prognostic Nuclear Features
Oncotopix® Discovery
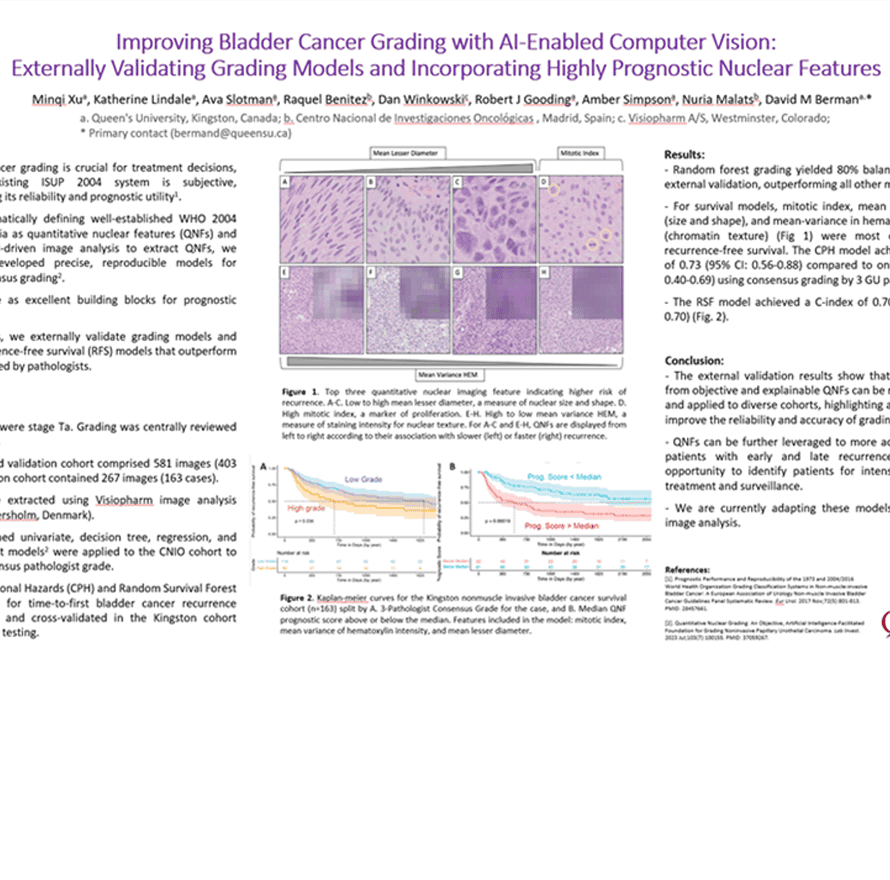
Improving Bladder Cancer Grading with AI-Enabled Computer Vision Externally Validating Grading Models and Incorporating Highly Prognostic Nuclear Features
Originally presented at USCAP 2024
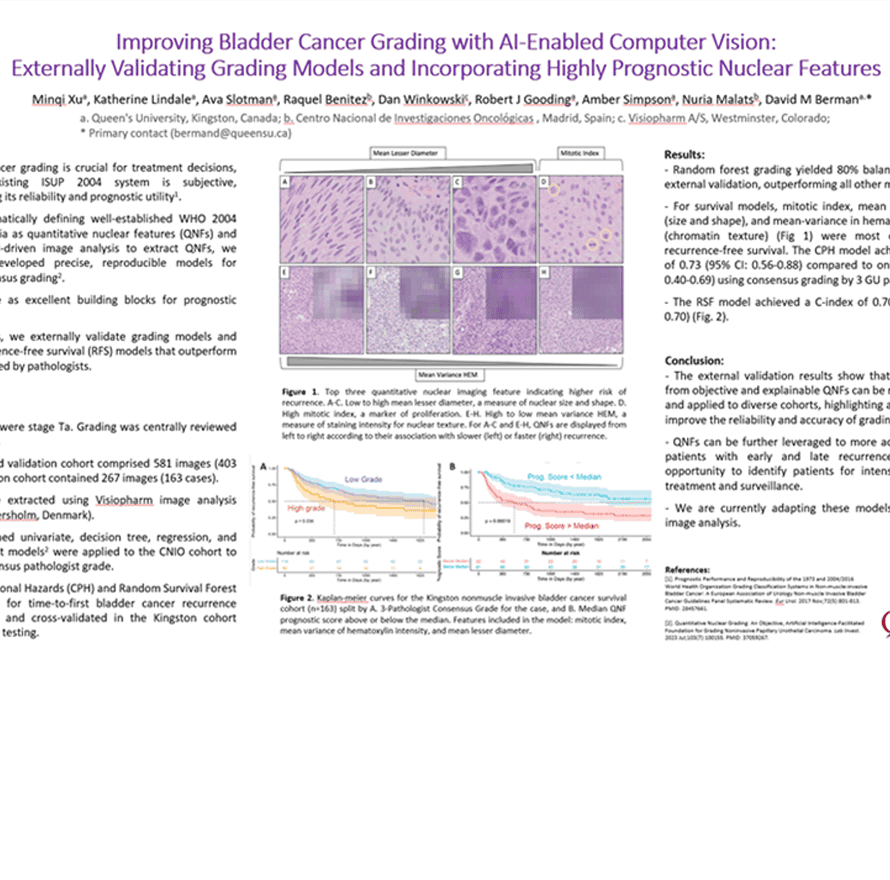
Description
- Bladder cancer grading is crucial for treatment decisions, but the existing ISUP 2004 system is subjective, compromising its reliability and prognostic utility.
- By mathematically defining well-established WHO 2004 grading criteria as quantitative nuclear features (QNFs) and employing AI-driven image analysis to extract QNFs, we previously developed precise, reproducible models for expert consensus grading.
- QNFs serve as excellent building blocks for prognostic classifiers.
- Using QNFs, we externally validate grading models and create recurrence-free survival (RFS) models that outperform grades assigned by pathologists.
Authors and institutions
Minqi Xua1, Katherine Lindalea1, Ava Slotmana1, Raquel Benitezb2, Dan Winkowskic3, Robert J Goodinga1, Amber Simpsona1, Nuria Malatsb2, David M Bermana1.
- Queen’s University, Kingston, Canada
- Centro Nacional de Investigaciones Oncológicas , Madrid, Spain
- Visiopharm A/S, Westminster, Colorado;