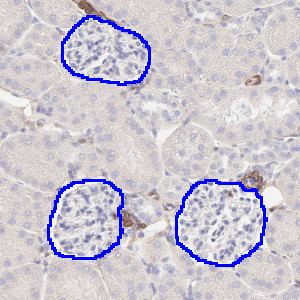
Segmented glomeruli in kidney samples stained with aSMA.
#10145
Developed for automatic segmentation of glomeruli in IHC stained kidney tissue
Glomeruli are the filtering units of the kidney. They are located at the beginning of nephrons and consist of a bundle of capillaries. This APP automatically identifies and segments glomeruli in kidney samples stained with various biomarkers including CD68, COL1A1, COLIV, synaptopodin, nephrin, WT1 and/or aSMA. It utilizes articifical intelligence (AI)/deep learning for a robust segmentation.
Quantitative Output variables
The output variable obtained from this protocol is:
Workflow
Step 1: Load an image
Step 2: Load and run the APP “10145 – IHC, Glomeruli Detection, AI”
Methods
The architectural structure of the deep learning network is a U-Net which is popular for medical image segmentation. The neural network uses a cascade of layers of nonlinear processing units for feature extraction and transformation, with each successive layer using the output from the previous layers as input. U-Net uses an encoder-decoder structure with a contracting path and an expansive path. For more information of the network architecture, see [1].
Staining Protocol
There is no staining protocol available.
Additional information
To run the APP, a NVIDIA GPU with minimum 4 GB RAM is required.
Keywords
Immunohistochemistry, IHC, Kidney, Glomerulus, Glomeruli, Artificial Intelligence, AI, Deep Learning, Image Analysis, Digital Pathology
References
LITERATURE
1. Ronneberger, O. et al. U-Net: Convolutional Networks for Biomedical Image Segmentation. International Conference on Medical Image Computing and Computer-Assisted Intervention, 2015, 234-241, DOI.