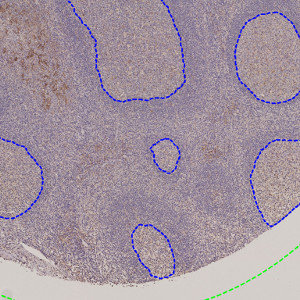
Outlined germinal centers (blue) on TMA core.
#10163
Developed for outlining germinal centers in tonsils
Tonsil tissue may be used as positive/negative controls for PD-L1 staining. Because the PD-L1 expression varies significantly between the tissue compartments, it is of interest to differentiate between tissue regions before evaluating the PD-L1 expression.
This APP automatically outlines germinal centers in tonsils stained for PD-L1 and is intended as an accessory APP to help isolate regions for further analysis.
Quantitative Output variables
The output variables obtained from this protocol are:
Workflow
Step 1: Load and run the APP 01 Germinal Center Detection
Methods
The APP was developed using the using the DeepLabv3+ neural network available with Author™ AI. The neural network uses a cascade of layers of nonlinear processing units for feature extraction and transformation, with each successive layer using the output from the previous layer as input. DeepLabv3+ uses an encoder-decoder structure with atrous spatial pyramid pooling (ASPP) that is able to encode multi-scale contextual information by probing the incoming features with filters or pooling operations at multiple rates and multiple effective field-of-views. This means that instead of using step-wise upsampling blocks to incorporate features from different levels, this network only needs two upsampling steps, i.e. it is faster to train and analyze than e.g. the U-Net. All of this also means that the decoder module can refine the segmentation results along the object boundaries more precisely. For more information on the network architecture, see [1].
Staining Protocol
There is no staining protocol available.
Additional information
To run the APP, a NVIDIA GPU with minimum 4 GB RAM is required.
Keywords
Tonsil, Germinal Center, PD-L1, Quality Control, AI, Deep Learning
METHODS
References
LITERATURE
1. Chen, L., et. al., Encoder-decoder with atrous separable convolution for semantic image segmentation, Proceedings of the European conference on computer vision (ECCV) 2018, 801-818, DOI