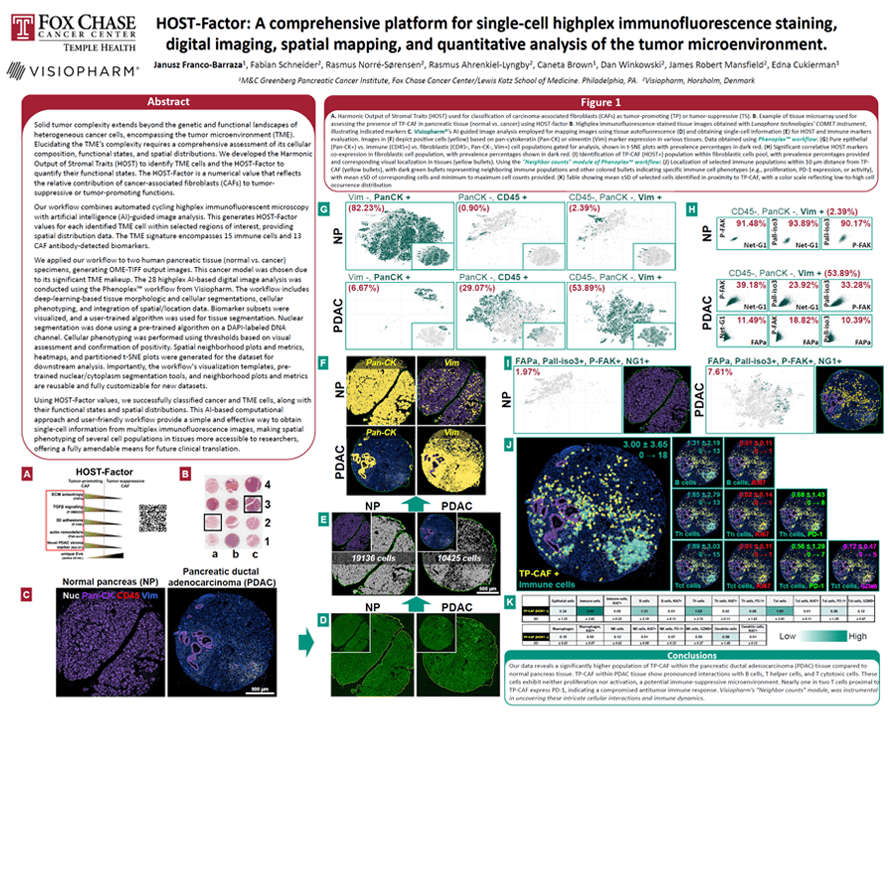
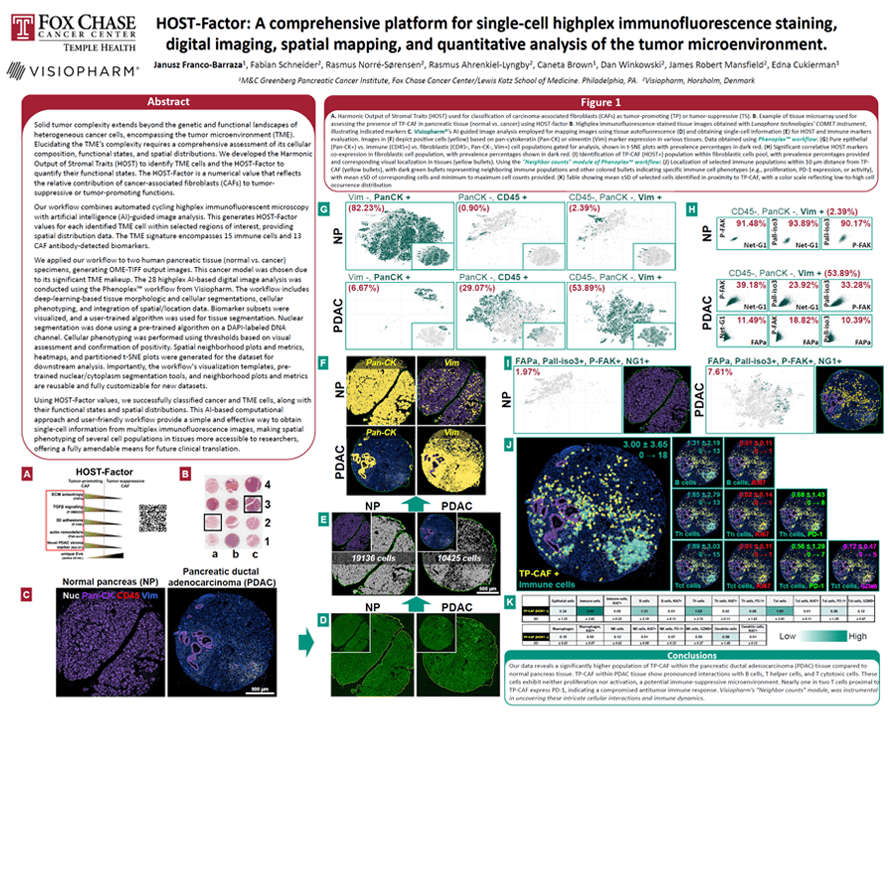
Solid tumor complexity extends beyond the genetic and functional landscapes of heterogenous cancer cells, encompassing the tumor microenvironment (TME). Elucidating the TME’s complexity requires a comprehensive assessment of its cellular composition, functional states, and spatial distributions. We developed the Harmonic Output of Stromal Traits (HOST) to identify TME cells, and the HOST-Factor to quantify their functional states. The HOST-Factor is a numerical value that reflects the relative contribution of cancer-associated fibroblasts (CAFs) to tumor-suppressive or tumor-promoting functions.
Our workflow combines automated cycling highplex immunofluorescent microscopy with artificial intelligence (AI)-guided image analysis. This generates HOST-Factor values for each identified TME cell within selected regions of interest, providing spatial distribution data. The TME signature encompasses 15 immune cells and 14 CAF antibodydetected biomarkers.
We applied our workflow to two human pancreatic tissue (normal vs. cancer) specimens, generating OME-TIFF output images. This cancer model was chosen due to its significant TME makeup. The 28 highplex AI-based digital image analysis was conducted using the Phenoplex workflow from Visiopharm. The workflow includes deep-learning-based tissue morphologic and cellular segmentations, cellular phenotyping, and integration of spatial/location data. Biomarker subsets were visualized, and a user-trained algorithm was used for tissue segmentation. Nuclear segmentation was done using a pre-trained algorithm on a DAPI-labeled DNA channel. Cellular phenotyping was performed using thresholds based on visual assessment and confirmation of positivity. Spatial neighborhood plots and metrics, heatmaps, and partitioned t-SNE plots were generated for the dataset for downstream analysis. Importantly, the workflow’s visualization templates, pretrained nuclear/cytoplasm segmentation tools, and neighborhood plots and metrics are reusable and fully customizable for new datasets.
Using HOST-Factor values, we successfully classified cancer and TME cells, along with their functional states and spatial distributions. This AI-based computational approach and user-friendly workflow provide a simple and effective way to obtain single-cell information from multiplex immunofluorescence images, making spatial phenotyping of several cell populations in tissues more accessible to researchers, offering a fully amendable means for future clinical translation.
Janusz Franco-Barraza1, Fabian Schneider2, Rasmus Norré-Sørensen2, Rasmus Ahrenkiel-Lyngby2, Caneta Brown1, Dan Winkowski2, James Robert Mansfield2, Edna Cukierman1
1) M&C Greenberg Pancreatic Cancer Institute, Fox Chase Cancer Center/Lewis Katz School of Medicine. Philadelphia, PA.
2) Visiopharm, Hørsholm, Denmark