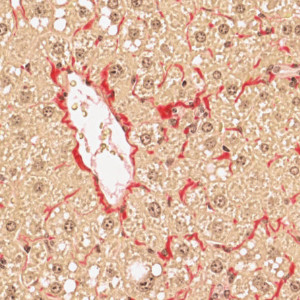
PSR stained liver tissue with a central vein.
#10156
DEVELOPED TO SUPPORT THE STAGING OF FIBROSIS FOR NAFLD AND NASH USING PSR IN RODENTS
In the development of medical knowledge within non-alcoholic fatty liver disease (NAFLD) and the more progressive form non-alcoholic steatohepatitis (NASH), it has been identified that biopsy evaluation is important for NAFLD treatment [1]. The condition is confirmed using the histopathological NAFLD Activity Score (NAS), which grades the severity of steatosis, hepatocellular ballooning degeneration, and inflammation. In addition, fibrosis is evaluated using a separate validated staging system. However, histopathological disease scoring systems are subjective and prone to inter- and intra-observer variation [2]. This Animal Research APP uses a deep learning-based strategy to obtain an accurate and objective method for scoring fibrosis in mouse models of NAFLD and NASH
This APP implements the Brunt fibrosis staging system [3] adapted for mouse models using a Picrosirius Red (PSR) stained section6. The work of this APP has been published as an abstract and as a poster on 17th European Congress of Toxicologic Pathology (ESTP 2019) and at the American Association for the Study of Liver Diseases 2019 (AASLD) [4].
For research use only. Not for use in diagnostic procedures.
Quantitative Output variables
The output variables obtained from this APP are:
• Fibrosis score: A score in the interval [0,1,2,3] using the perisinusoidal, periportal and bridging fibrosis.
Workflow
The APP contains six protocols:
Step 1: Load and run the APP “01 Tissue detection” for tissue detection
Step 2: Load and run the APP “02 Central vein & portal triad detection” for central vein and portal triad detection
Step 3: Load and run the APP “03 Fibrosis in periportal space” to detect fibrosis in periportal space
Step 4: Load and run the APP “04 Fibrosis in perisinusoidal space” to detect fibrosis in perisinusoidal space.
Step 5: Load and run the APP “05 Bridging in perisinusoidal space” to detect bridging fibrosis in perisinusoidal space.
Step 6: Load and run the APP “06 Fibrosis score” to calculate the score using the quantification of the previous APPs.
Methods
The APP contains five protocols:
• “01 Tissue detection”: Liver tissue is outlined using threshold-based image analysis (IA).
• “02 Central vein and portal triad detection”: A deep learning network DeepLabv3+ [5] available with AuthorTM AI is used to detect central veins and portal triads. Since DeepLabv3+ utilizes atrous spatial pyramid pooling, this network architecture is powerful to segment objects when contextual information is important.
• “03 Fibrosis in periportal space”: Fibrosis is calculated in the periportal space using traditional image analysis. .
• “04 Fibrosis in perisinusoidal space”: Fibrosis is calculated in the perisinusoidal space using traditional image analysis.
• “05 Bridging in perisinusoidal space”: Bridging fibrosis is detected in the perisinusoidal space using using traditional image analysis.
• “06 Fibrosis score”: Calculates the fibrosis score using the quantification of perisinusoidal, periportal and bridging fibrosis.
Additional information
To run the APP, a NVIDIA GPU with minimum 4 GB RAM is required.
The APP utilizes the Visiopharm Engine™ AI and Viewer software modules, where Engine™ AI offers an execution platform to expand processing capability and speed of image analysis. The Viewer allows a fast review together with several types of image adjustment properties e.g. outlining of regions, annotations and direct measures of distance, curve length, radius, etc.
By adding the Author™ AI module the APP can be customized to fit other purposes. Author™ AI offers a comprehensive and dedicated set of tools for creating new fit-for-purpose analysis APPs, and no programming experience is required.
Keywords
Scoring, Non-alcoholic Fatty Liver Disease, NAFLD, Non-alcoholic Steatohepatitis, NASH, Deep Learning, AI, Picrosirius Red, PSR, Mouse, NAFLD Activity Score, NAS, Digital Pathology
References
[1] Spengler, E. K. & Loomba, Rohit. Recommendations for diagnosis, referral for liver biopsy, and treatment of nonalcoholic fatty liver disease and nonalcoholic steatohepatitis. Mayo Clinic Proceedings, 2015, 90 (9), 1233–1246. https://doi.org/10.1016/j.mayocp.2015.06.013
[2] Pournik, O., et al. Inter-observer and intra-observer agreement in pathological evaluation of non-alcoholic fatty liver disease suspected liver biopsies. Hepatitis Monthly, 2014, 14 (1), 3–6. https://doi.org/10.5812/hepatmon.15167.
[3] Brunt, E. M., et al. Nonalcoholic steatohepatitis: A proposal for grading and staging the histological lesions, 1999, 94 (9), 2467–2474. DOI: 10.1111/j.1572-0241.1999.01377.x
[4] Overgaard, A., et al. Histopathological scoring of non-alcoholic fatty liver disease using deep learning. Hepathology. 2019, 70 (S1), abstract no.1754. https://aasldpubs.onlinelibrary.wiley.com/doi/10.1002/hep.30941
[5] Chen, L., et al. Encoder-decoder with atrous separable convolution for semantic image segmentation. Proceedings of the European conference on computer vision (ECCV) 2018, 801–181, arXiv:1802.02611