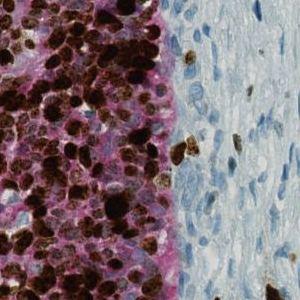
One field of view of the original image at 20X.
#10046
Cervical Cancer is malignant neoplasm that forms in the cells origination in the cervix uteri. It is a slow-growing cancer typically having no symptoms in the early stages. As the cancer progress to a more advanced stage abnormal vaginal bleeding is the most common symptom. Diagnose of cervical cancer, or cervical dysplasia, is based on an examination of cervical biopsies typically using a Pap smear test.
The Ki-67 protein is associated with cellular proliferation, and the protein is present in the nucleus of all cells that are in the active phase of the cell cycle, but absent in resting cells, see [1]. The cell proliferation rate can be assessed by Ki67-immunohistochemical (IHC) staining, and this can be correlated to the tumor grade and the clinical course for the patient, see [2].
p16 is a tumor suppressor protein and plays and an important role in regulating the cell cycle. Increased expression of the protein reduces the production and division of stem cells and is associated with an increased risk of a wide range of cancer. Detection of p16 is particularly used in the classification of cervical dysplasia and in the differential diagnosis of the two main types: cervical adenocarcinoma versus endometrial adenocarcinoma.
This APP can be used to find the nuclei within a tumor region for double Ki-67+p16 staining. The protocol will provide the proliferations rate (area and number) of nuclei within the tumor regions. No manual outlined of Region of Interest (ROI) is necessary since the protocol is able to outline the tumor regions based on the double staining (Ki-67+p16). Thus only an overall ROI is required.
Quantitative Output variables
The output variables of the protocol include:
Methods
The first image processing step involves a segmentation of all nuclei. Nuclei are identified by assigning a label probability to all pixels in the image, resulting in a label probability image. The label probability image is found by an intensity dictionary – a dictionary with small image patches. The intensity dictionary can be coupled to a label dictionary from which the label probability image is obtained. Based on this image, segmentation of nuclei can be done by choosing the most probable label in each pixel, see [3]. A method for nuclei separation which is based on shape, size and nuclei probability is used, employing a fully automated watershed-based nuclei segmentation technique. The method is an extension of the method proposed by Jung and Kim, see [4], where an h-minima transform is used before applying the watershed. The nuclei are subsequently divided into positively stained nuclei and negatively stained nuclei. In addition, the cytoplasm surrounding the nuclei is found and segmented into tumor areas and non-tumor areas depending on the color (see FIGURE 2). Next, nuclei surrounded by cytoplasm belonging to stromal tissue a tumor region are segmented. Furthermore, a post-processing step is applied, to separate nuclei that are too large. The image obtained after post-processing (see FIGURE 3) is used to determine the output variables.
Staining Protocol
There is no staining protocol available.
Keywords
Ki67, Ki-67, p16, double staining, proliferation index, uterine cervix, quantitative, digital pathology, image analysis.
References
LITERATURE
1. T. Scholzen et al., The Ki-67 protein: from the known and the unknown, J. Cell Physiol. 2000, 182 (3), 311-22, DOI
2. NordiQC: Epitope Ki67. (Last updated/Accessed: 23/08-2019).
3. Dahl, A.L. et. al., Learning Dictionaries of Discriminative Image Patches, British Machine Vision Conference 2011, DOI
4. Jung, C. et. al., Segmenting Clustered Nuclei Using H-minima Transform-Based Marker Extraction and Contour Parameterization, IEEE Transactions on Biomedical Engineering 2010, 57 (10), 2600-2604, DOI