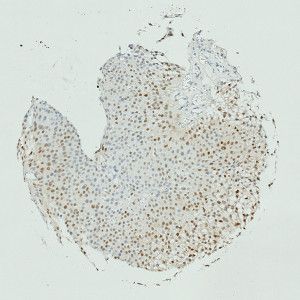
Image of TMA core with bladder tumor tissue after staining by IHC for CyclinD1.
#10013
Cyclin D1 is often deregulated in cancer and is a biomarker of cancer phenotype and disease progression, see [1]. Cyclin D1 has been shown to regulate the expression of genes that are involved in DNA replication and the DNA damage checkpoint, and it has also been shown to interact with DNA damage response proteins, see [2], [3]. Cyclin D1 overexpression is associated with shorter patient survival in many cancers and has been shown to associate with increased metastasis, see [4], [5]. The ability of Cyclin D1 to activate the cyclin-dependent kinases CDK4 and CDK6 is an extensively documented mechanism for its oncogenic actions and could therefore be a possible therapeutic target in future medicine, see [1].
This APP can be used for quantifying the nuclear expression of Cyclin D1. The approach to calculating expression used here, was demonstrated to have a very high correlation to manual scoring in a study involving 300 patients (R2 = 0.85), (publication pending).
Quantitative Output variables
The output variables obtained from this protocol include:
Methods
The method used for computing the Cyclin D1 expression is started by detecting nuclei using a novel pattern recognition method adapted from Dahl et al, see [6], which is followed by a step that separates adjacent nuclei, see [7]. The detected nuclei are classified as either positive or negative based on a computation of DAB intensity, obtained using color deconvolution.
This leads to the calculation of the positive area fraction, as the area of positive nuclei divided by the total area of nuclei (within the outlined ROI). This is followed by the measurement of the average DAB intensity across all positive nuclei, which is subtracted from 255, to associate higher values with a high staining intensity, which is more intuitive. The expression is then calculated by multiplying the intensity with the area fraction.
The actual implementation of the pattern recognition allows the user to choose settings for nuclear detection sensitivity and classification into positive and negative, in order to account for differences in local staining protocols.
It should be noted that this approach to quantifying biomarker expression is vulnerable to variations in protocols for tissue preparation (fixation time, tissue thickness, reagents, auto-stainer type settings, etc.). Using this APP for TMA study designs should be quite robust, for the cores within the TMA.
This APP requires manual outlining of tumor regions.
Keywords
Cyclin D1, bladder cancer, metastasis, immunohistochemistry, image analysis, quantitative, digital pathology.
References
USERS
This APP was developed for, and validated by, Niels Fristrup MD and Dr. Lars Dyrskjøt Andersen, Center for Molecular Clinical Cancer Research Department of Molecular Medicine (MOMA) Aarhus University Hospital.
LITERATURE
1. Musgrove, E.A., et. al. Cyclin D as a therapeutic target in cancer, Nat Rev Cancer 2011, 11 (8), 558-72, DOI
2. Jirawatnotai, S., et. al. A function for cyclin D1 in DNA repair uncovered by protein interactome analyses in human cancers, Nature 2011, 474 (7350), 230-4, DOI
3. Li, Z., et. al. Alternative cyclin D1 splice forms differentially regulate the DNA damage response, Cancer Res. 2010, 70 (21), 8802-11, DOI
4. Jares, P., et. al. Genetic and molecular pathogenesis of mantle cell lymphoma: perspectives for new targeted therapeutics, Nature Rev. Cancer 2007, 7 (10), 750-62, DOI
5. Thomas, G.R., et. al. Molecular predictors of clinical outcome in patients with head and neck squamous cell carcinoma, Int. J. Exp. Pathol. 2005, 86 (6), 347-363, DOI
6. Dahl, A.L., et. al. Learning Dictionaries of Discriminative Image Patches, British Machine Vision Conference 2011, DOI
7. Jung, C., et. al. Segmenting Clustered Nuclei Using H-minima Transform-Based Marker Extraction and Contour Parameterization, IEEE Transactions on Biomedical Engineering 2010, 57 (10), 2600-4, DOI