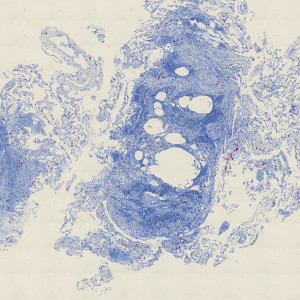
CD3 stained lung tissue.
#10011
Over the last two decades, lung transplantation has evolved as an accepted treatment of end-stage pulmonary failure unresponsive to other therapy, see [1]. However, long-term outcome is still poor – mainly due to chronic allograft rejection with fibrous obliteration of the small airways. The immunological background for this condition is not well understood, but acute rejection is a known risk factor for later development of chronic rejection, see [2]. The study of inflammation during acute rejection episodes might therefore offer new insights into the mechanisms leading to chronic rejection. Regulatory T lymphocytes (Tregs) are a subset of T cells known to suppress a wide range of immune responses, see [3]; they are considered pivotal for the induction of tolerance to donor antigens in different human allografts. Therefore it could be of relevance to study the number of Tregs as a fraction of the total number of T cells in lung biopsies and compare it with the patient’s rejection status and lung function. With immunohistochemistry CD3 positive T cells can be identified, but manual cell counting of these is both difficult and time consuming because of the very large number of cells.
With the APP “CD3, Lung Transplantation” automated and fast quantification of the CD3 positive T cells can be obtained.
The APP automatically identifies the CD3 positive areas on the slide and outputs amongst others the CD3 positive ratio.
Auxiliary APPs
Auxiliary APPs are used for additional process steps, e.g. finding Region of Interest (ROI).
ROI DETECT
The auxiliary APP ’01 ROI Detect’ can be used to automatically detect the region of interest (ROI). The APP utilizes a Bayesian classifier to detect the tissue areas of interest (see FIGURE 1 and 2). It might, sometimes be needed to manually remove areas of no interest subsequently to e.g. exclude normal and necrotic tissue.
REMOVE BLOOD
The auxiliary APP ’02 Remove Blood’ can be used to automatically detect the lung tissue containing bleed outs and exclude these from the study. The APP utilizes a Bayesian classifier to detect variation in the lung tissue and exclude regions that are likely to be blood. It might, sometimes be needed to manually remove areas of no interest or include areas of interest subsequently.
Quantitative Output variables
The output variables obtained from this protocol include:
Workflow
Step 1: Load auxiliary APP for tissue detection ’01 ROI detect’ and run on the image to process. This outlines the tissue with a Region Of Interest (ROI) and saves time in the subsequent analysis steps.
Step 2 (Optional): Load auxiliary APP ’02 Remove Blood’ and run on the image to remove artifacts stemming from tissue with bleed out, from the analysis. These will subseqyently not be included in the calculations.
Step 3 (Optional): Manually edit the ROI, using the layer drawing tool, if you wish to remove or add additional areas to the analysis.
Step 4: Load APP quantification protocol ’03 Analyze’ and run on the images to process. The third image processing step detects the CD3 positive stains.
Methods
The first image processing step involves the identification of lung tissue and the removal of lung tissue which contains blood (see FIGURE 1 and 2). To quantify the tissue, a color deconvolution of CD3 and Hematoxylin is used to segment out the red CD3 positively stained areas from the blue lung tissue (see FIGURE 3 and 4). It is important to exclude major airways and other non-lung tissue objects. Based on the identification of the CD3 positive areas it is possible to calculate a CD3 positive expression value and the CD3 positive area fraction.
Note on counting: Analysis of full virtual slides takes place in a tile-by-tile fashion. There is a risk of counting the CD3 positive T cells twice (or more) in cases where the cells are intersecting with neighboring tile boundaries. Using unbiased counting frames, see [4], this can be avoided. With the size and density of the CD3 positive T cells the application of this principle could make a difference of approx. 2%.
For this specific APP the processing overhead of applying unbiased counting frames is significant, so by default the counting frames have been disabled, but can be enabled for higher counting precision.
Staining Protocol
There is no staining protocol available.
Keywords
Lung transplantation, acute rejection, chronic rejection, CD3, T lymphocytes, quantitative, digital pathology, image analysis.
References
USERS
This APP was developed for, and validated by, Dr. Ben Vainer MD and Dorrit Krustrup, Molecular Pathology Section, Department of Biomedical Sciences, University of Copenhagen.
LITERATURE
1. Burton, C.M., et. al. Long-term survival after lung transplantation depends on development and severity of bronchiolitis obliterans syndrome, J. Heart Lung Transplant 2007, 26 (7), 681-6, DOI
2. Burton, C.M., et. al. Acute rejection is a risk factor for bronchiolitis obliterans syndrome independent of post-transplant baseline FEV1, J. Heart Lung Transplant 2009, 28 (9), 888-93, DOI
3. Vignali, D.A. How regulatory T cells work, Nat Rev Immunol 2008, 8 (7), 523-32, DOI
4. Howard, C.V., Reed, M.G. (2005). Unbiased Stereology: Three-dimensional measurement in microscopy. QTP Publications.